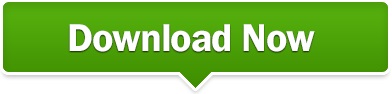
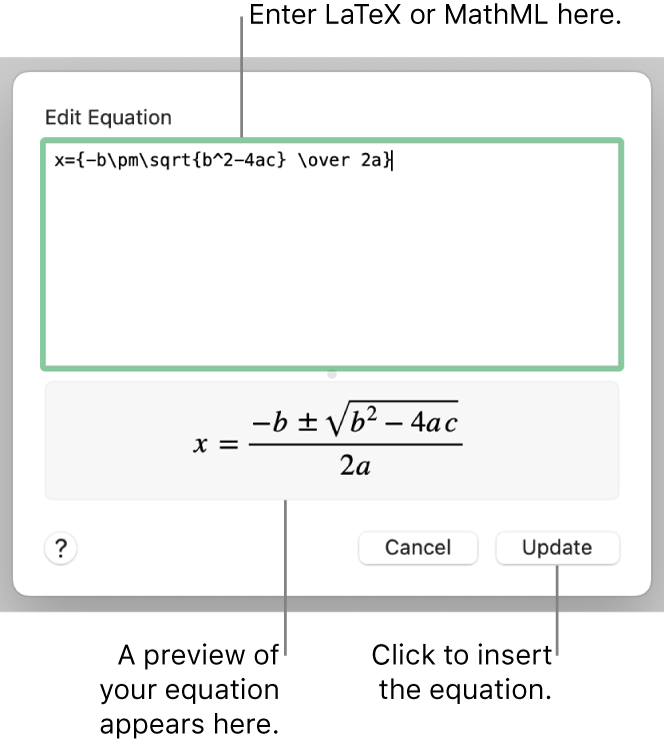
These three approaches give similar results, but the last ones will be preferred when samples are small. The median residual lifetime is one of the key results of the Kaplan-Meier analysis as it allows to evaluate the time remaining for half of the population to "fail".Ĭonfidence interval for the Kaplan-Meier analysis functionĬomputing confidence intervals for the survival function can be done using three different methods:


This table displays the various results obtained from the analysis, including: Results for the Kaplan-Meier analysis in XLSTAT Kaplan-Meier table Random: the time when a censoring occurs is independent of the survival time.Type II: the study is continued until n events have been recorded.Progressive type I: all individuals are censored at the same date (for example, when the study terminates).Simple type I: all individuals are censored at the same time or equivalently individuals are followed during a fixed time interval.There are four different types of independent censoring: Second, the censoring must be independent: if you consider two random individuals in the study at time t-1, if one of the individuals is censored at time t, and if the other survives, then both must have equal chances to survive at time t. The Kaplan-Meier method requires that the observations are independent. Exact censoring: when an event is reported at time t=t (i), we know that the event occurred exactly at t=t (i).Interval censoring: when an event is reported at time t=t (i), we know that the event occurred during.Right censoring: when an event is reported at time t=t (i), we know that the event occurred at t * t (i), if it ever occurred.Left censoring: when an event is reported at time t=t (i), we know that the event occurred at t * t (i).There are several types of censoring of survival data: Censoring data for Kaplan-Meier analysis Types of censoring These results can later be used to model the survival curves and to predict probabilities of failure. Tests can be performed to check if the survival curves have arisen from identical survival functions. For example, it can be of interest to compare the survival times of two samples of the same product produced in two different locations. The Kaplan-Meier analysis allows you to compare populations, through their survival curves. The first type of data is usually called failure data, or event data, while the second is called censored data. There are three main reasons why a population of individuals or products may evolve: some individuals die (products fail), some other go out of the surveyed population because they get healed (repaired) or because their trace is lost (individuals move from location, the study is terminated, among other reasons). This technique is mostly applied to survival data and product quality data. Kaplan-Meier analysis is used to analyze how a given population evolves with time. Kaplan-Meier analysis, which main result is the Kaplan-Meier table, is based on irregular time intervals, contrary to the life table analysis, where the time intervals are regular. Kaplan-Meier analysis allows you to quickly obtain a population survival curve and essential statistics such as the median survival time. The life table analysis method was developed first, but the Kaplan-Meier method has been shown to be superior in many cases. The Kaplan-Meier method, also called product-limit analysis, belongs to the descriptive methods of survival analysis, as does life table analysis.
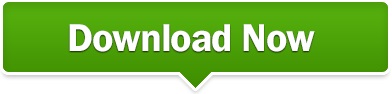